New technologies require a macro-triggering event to make the jump from more restrictive to more mainstream use.
- The web browser did this for the Internet, moving us from restricted private clouds to the open public cloud (World Wide Web).
- ChatGPT did this for AI, moving us from more exclusive use by large companies to more accessible use by anyone.
Much like the web browser democratized the Internet, ChatGTP democratized AI.
While it’s inevitable that such technologies go on to change the world, this change doesn’t come instantly but in stages, and we’re currently somewhere between stage 2 and stage 3.
Much like we had the dot com crash before the boom, we should brace for the dot ai crash (already started) before the plateau of productivity.
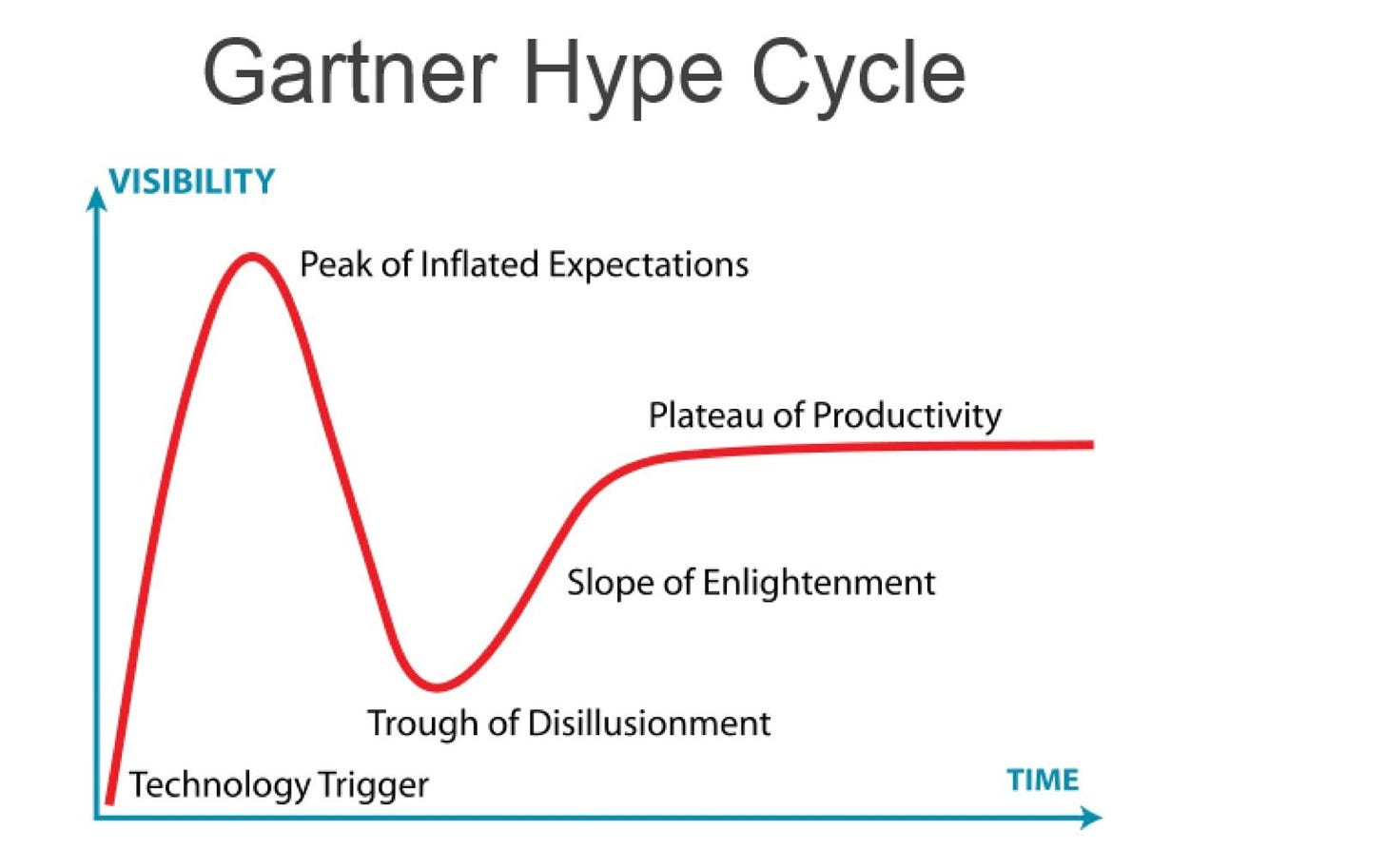
How early-stage entrepreneurship will be taught and practiced will inevitably change, too, and the time for tinkering is now.
A use case I’m particularly interested in is how early-stage entrepreneurship will be practiced and taught. In this issue, I’ll share some of my early experiments with AI and thoughts on where it’s perfectly positioned to help and where it isn’t.
The peak of inflated expectations
Shortly after the launch of ChatGPT, it didn’t take long for several AI-driven startup idea generators to pop up.
With a few inputs, you get a completed Lean Canvas, a pitch deck, marketing collateral, and even some starting code and landing pages.
But,
Idea validation > > Idea generation
I toyed with a few, and the results were lackluster.
While LLMs will continue to improve on the breadth of their knowledge, I’m still skeptical about LLM use for “good” idea generation for the following reasons:
1. Going from zero to one requires non-linear or lateral thinking grounded in discovery
All startup ideas that work sound crazy at first because they go against status quo thinking. They are often built upon a combination of lateral or non-linear thinking coupled with unique insights or secrets.
While one can dial up on crazy by specifying more creativity in reasoning (with LLM temperature), getting to real insights requires doing the hard work of customer problem discovery.
Breakthrough insights, by definition, lay hidden below the surface and can’t simply be inferred from publicly available knowledge.
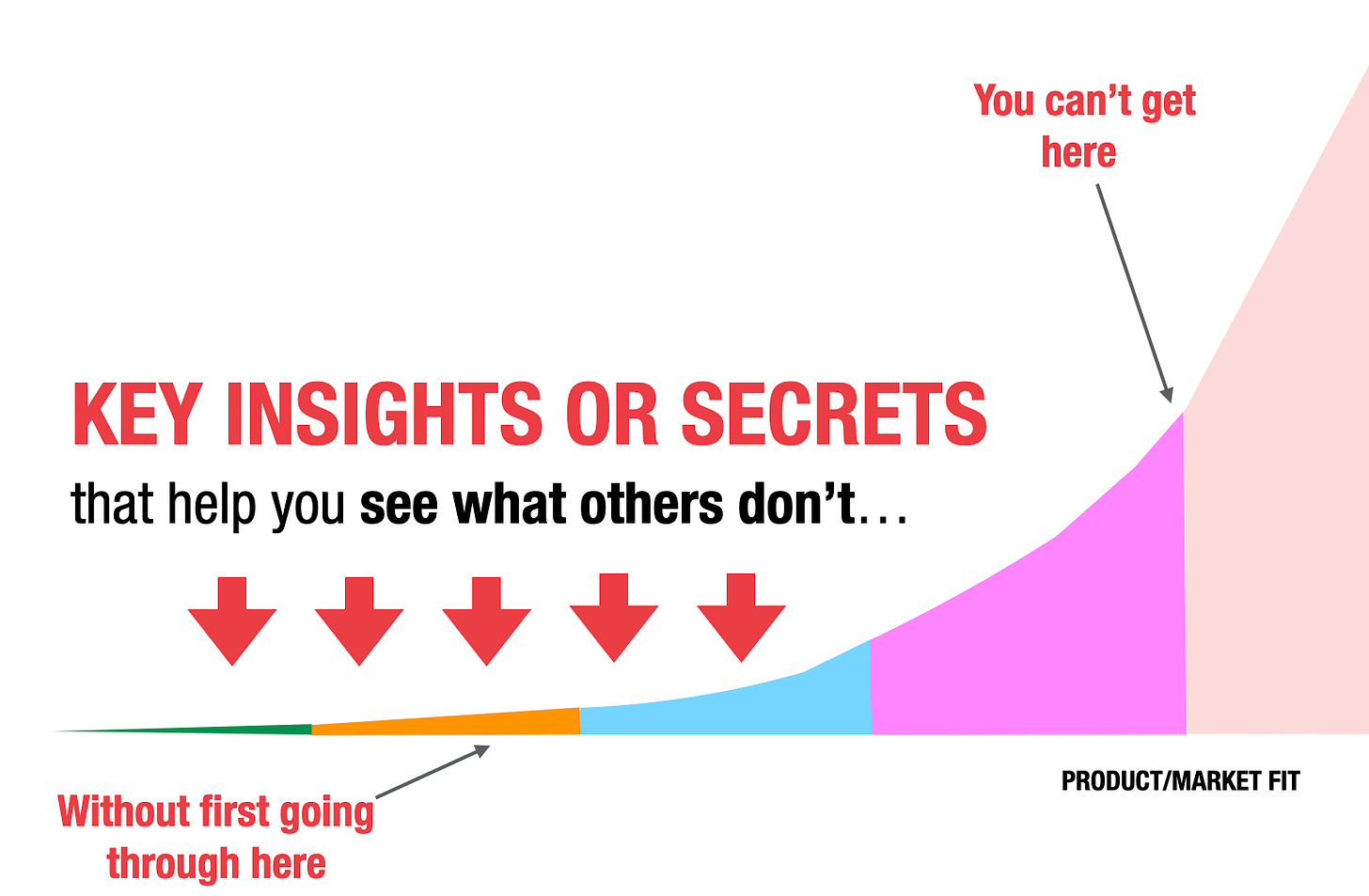
2. Continuous critical thinking is still required to turn even a good idea into a working business model
Another real danger with LLMs is over-reliance on pseudo-intelligent-sounding LLM responses where we get lazy and swap founder cognitive biases for machine-generated biases. And we’re back to executing a flawed plan.
There is no substitute for critical thinking.
While I’m not betting the farm on the next big idea coming from generative AI, I am intrigued and encouraged by the next set of use cases.
Slope of enlightenment
I believe that the more immediate potential for LLMs is helping early-stage teams to
- Learn faster through simulations and
- Make better evidence-based decisions.
We don’t want to dial up LLM creativity in both these use cases but down — favoring predictability in reasoning over hallucinations.
1. Faster learning through simulations
This resembles how chess players use machine-run game simulations to learn faster.
Given an input model, like Lean Canvas, and a series of instruction-based stress-best recipes, it is possible to highlight and suggest refinements to help founders improve their critical thinking.
2. Evidence-based decision making
Making good decisions with imperfect information is what separates successful founders from others. This is an area I find most prime for picking.
The specific use case I’m testing is shortening the cycle time from customer problem discovery interviews to actionable insights (finding problems worth solving). Not only is this process currently prone to extreme founder bias, but it’s also the most critical step toward building something customers want.
In theory, all the evidence is either in the interview transcripts or not. So, asking the LLM to separate fact from fiction (and cite sources in the transcript) is the perfect way to keep insights in check.
Early experiments combining “interview transcripts + LLM training on customer forces stories + similarity clustering” have been promising. I’ll share the results in a future issue.
Plateau of productivity
We are still in the early stages of a new era. I am rationally optimistic that LLMs, combined with the right balance of fine-tuning and feedback loops, have immense potential to usher in the next wave of entrepreneurship, but the path isn’t a straight shot. It never is.